Welcome to the Feature Store newsletter brought to you by Logical Clocks, where we in a monthly cadence will highlight the latest news, events, and insights as we help make companies successful in their machine learning transformation journey and empower businesses to be applied-AI model-driven companies.
The ‘Feature Store’ is an emerging concept in data architecture that is motivated by the challenge of taking ML applications into production. Technology companies like Uber and Gojek have published popular reference architectures and open source solutions, respectively, for ‘Feature Stores’ that address some of these challenges.
The concept of Feature Stores is nascent and we’re seeing a need for education and information regarding this topic. Most innovative products are now driven by machine learning. Features are at the core of what makes these machine learning systems effective. But still, many challenges exist in the feature engineering life-cycle. Developing features from big data is an engineering heavy task, with challenges in both the scaling of data processes and the serving of features in production systems.
A Feature Store will extend your data infrastructure to support ML-specific requirements and enable data science teams to;
- Track and share features between data scientists including a version-control repository
- Process and curate feature values while preventing data leakage
- Ensure parity between training and inference data systems
- Serve features for ML-specific consumption profiles including model training, batch and real-time predictions
- Accelerate ML innovation by reducing the data engineering process from months to days
- Monitor data quality to rapidly identify data drift and pipeline errors
- Empower legal and compliance teams to ensure compliant use of data
- Bridging the gap between data scientists and data & ml engineers
Other Feature Store business benefits apart from the cost and operational efficiencies mentioned above are:
- Lower total cost of ownership through automation and simplification
- Faster Time-To-Market for new model-driven products
- Improved model accuracy: the availability of features will improve model performance
- Improved data quality via data ->feature -> model lineage
A great starting point to learn more about the technical aspects of a Feature Store as a feature management platform for machine learning is our white paper. It will help you understand the concept of a Feature Store, online versus offline usage, technical integrations, ML-pipelines, and use cases.
Hopsworks Feature Store
Feature Stores in Production
In this, our first issue, we list all Feature Stores that run in production that we know about. If we missed one, let us know!
- Michelangelo (Uber): InfoQ Talk 2019
- Hopsworks (Logical Clocks): Bay Area AI Talk 2019
- Feast (GoJek): HasGeek TV Talk 2019
- Zipline (AirBnB): Spark/AI Summit 2019
- Metaflow (Netflix): MLOps 2019
- FBLearner (Facebook): TWiML Interview 2018
- Feature Factory (Databricks): Spark/AI Summit 2019
- Online Feature Store (Zomato): HasGeek TV Talk 2019
- Galaxy (PInterest): Slides from 2019
- SurveyMonkey Feature Store: Slides from 2020
- Comcast Feature Store: Spark Summit 2018
- Wix Feature Store: Wix Engineering Talk 2019
- Twitter Feature Store: MLOps 2019
Editors Picks
A great example of a feature store in production and how it improves “serviceability” in Gojek’s food delivery service GoFood, they aim to ensure that their need for a fresh, satisfying meal is met quickly. When dealing with large order volumes across 400,000+ merchant partners, they wanted to ascertain upfront whether we would be able to reliably service each order. A feature store is a key infrastructure element to making this happen
Read more
Very insightful explanation from Google about the role of feature stores for providing predictions from machine learning models, as well as techniques for minimizing the prediction serving latency of ML systems
Read more
MLOps with a Feature Store
If AI is to become embedded in the DNA of Enterprise computing systems, Enterprises must first re-align their machine learning (ML) development processes to include data engineers, data scientists and ML engineers in a single automated development, integration, testing, and deployment pipeline. This blog introduces platforms and methods for continuous integration (CI), continuous delivery (CD), and continuous training (CT) with machine learning platforms, with details on how to do CI/CD machine learning operations (MLOps) with a Feature Store. We will see how the Feature Store refactors the monolithic end-to-end ML pipeline into a feature engineering and a model training pipeline.
Read more
Webinar: Hopsworks Feature Store for Databricks
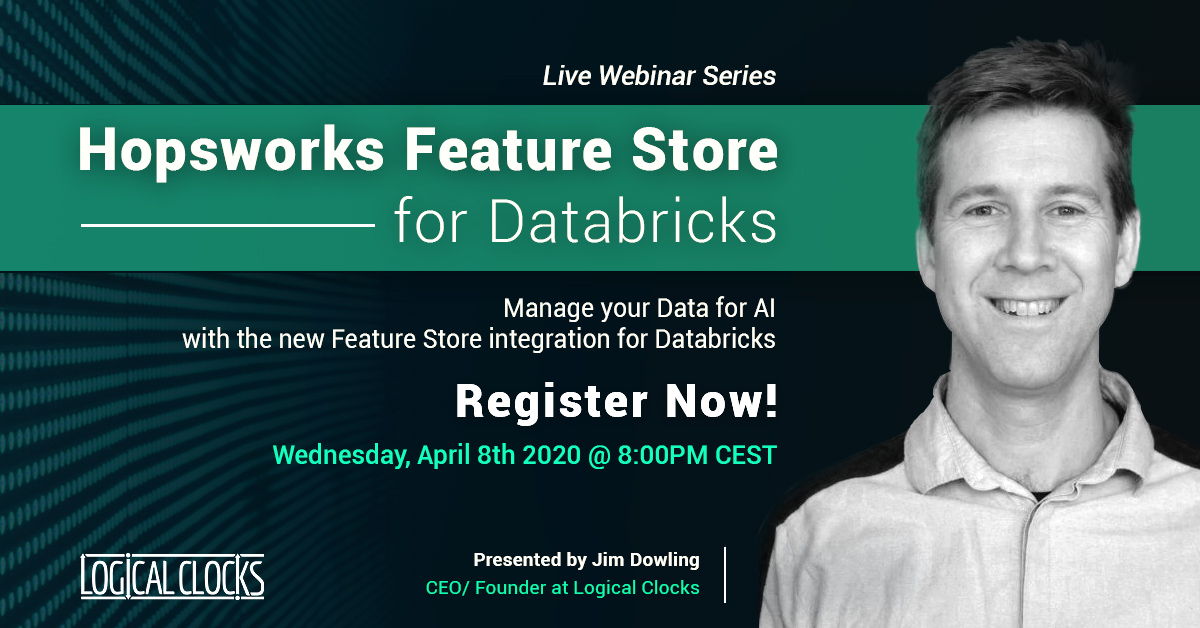
During this webinar we will introduce the concept of a Feature Store and how it helps manage data for AI. We will walk-through the Hopsworks Feature Store, introducing its concepts and how you can use it from Databricks for feature engineering, as a feature registry, for creating train/test datasets for ML, and as an online Feature Store to build feature vectors for online applications with low latency.